So, Google Translate is sexist. Or, at least, that’s the claim made in the following, viral tweet:


Before you jump to defend Google Translate by pointing out that this example is contrived, someone tried a much less contrived example and it still mangled it in the exact same way:

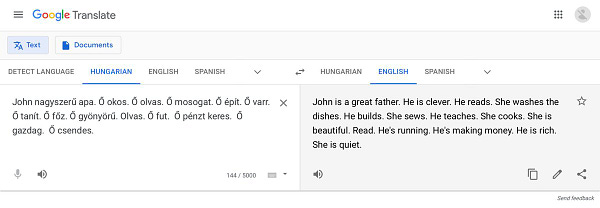
As Felix Salmon pointed out in a reply to my thread on this tweet, and I certainly agree with him, if a human translator produced the above, we’d say that translator was sexist.

I kept thinking about Felix’s point after our exchange, and one question still nagged at me: What does it mean to say that a machine translation (MT) model is “sexist”?
As I thought about this question, I unpacked it into a collection of further questions, which I’ll present below in the form of a dialogue.
A dialogue concerning machine translation
We join the dialogue in-progress, our participants having already begun to discuss the question of what it means to call Google Translate “sexist.”
Socrates: Wouldn’t calling an MT model “sexist” mean that the model’s training has encoded a bunch of sexist patterns in it, patterns that it’s then reproducing in the world via language?
Timaeus: Perhaps this is so, Socrates. And if it is indeed so, then is that also what it means for a human (brain) to be sexist? (I.e. that the person was “trained” on sexist inputs, and reproduces those patterns in the world?)
Critias: Certainly neither of you has the right of it, friends. For the model is simply a tool — like a turbocharged dictionary — and not a person against whom one can bring a charge of “sexism”.
Google Translate is a translation aide, not a translator. A human must take its output and further edit it to suit his audience. Thus the blame for any sexism in the resulting translation is on the human user of this tool, and not on the tool, itself.
Timaeus: But surely it’s possible, dear Critias, to say that a simple tool, like a dictionary, is objectively “sexist” if it contains sexist language. It follows, then, that we can call a tool “sexist” because its makers are sexist.
But regardless of whether we describe the tool itself as “sexist,” I think you’re correct to turn our attention to the matter of who is to blame. Indeed, I believe the real question we should all consider is: who do we blame for the translation tool’s sexism?
Socrates: Well said, Timaeus! In the case of a dictionary, we blame the authors. In the case of Google Translate, perhaps we should therefore blame Google. Might we conclude it is Google that is sexist, for they have produced a sexist “dictionary” (of sorts)?
Hermocrates: Certainly not, Socrates. Google is surely not to blame for producing a “dictionary” (as you say) that merely reflects the sexism found in everyday speech.
Is it not the responsibility of a dictionary (and a dictionary’s authors!) to faithfully represent the language as it is, and not as they wish it to be? It’s no fault of Google’s if our language is sexist, nor is it the fault of their model, but rather the fault lies with the interlocking systems of domination and oppression that structure the social context in which translation, itself an exercise of power...
Socrates: Slow your roll, Woke-ocrates! Before you lead us down that particular rabbit hole, I’d like to introduce a fact we have not yet considered: machine translation is increasingly being used in certain technical and bureaucratic applications as a replacement for a human translator. So it is not always acting not merely as a tool or translation aid, but in many cases, it acts as a standalone translator in its own right.
How then, are we to assign blame for the things our machines do in the world when they act — much as we do — out of a combination of past training, present prompts, and some expectations about the future? Is the blame with the parent, or with the child, or with the educational system, or with society?
Critias: If we allow our conversation to take this turn, Socrates — if we begin to consider the machine acting in the place of a human translator— then what of the recent debate around the Dutch translation of Amanda Gorman’s inaugural poem? A machine cannot be black, or female, and so on. So if we hold the machine to the same standard as Gorman’s Dutch translators insist on, we could never produce a machine translation!
Socrates: Then let us by no means hold to that standard, Critias. For if we did, then how would a future generation understand our own words after we’re long dead? How would some person thousands of years from now join this very conversation from across the ages?
It was at this point that I realized I had entered into the realm of the general philosophy and politics of translation. I spent years of my life (about ten) in graduate school, translating ancient texts (mostly Greek literature, but some Hebrew and a tiny bit of Aramaic), but I needed to call for backup. I needed professional help.
So I reached out to Art Goldhammer and asked him to help me think through some of these issues on a Zoom call. He graciously agreed, and the results were incredibly helpful. Our conversation helped me get unstuck in some important ways, and I hope it helps others, as well.
Politics of translation
Art and I know each other from the Internet, and it has been my pleasure to correspond with him periodically over the years on a variety of topics of mutual interest. His translation of Piketty’s Capital in the Twenty-First Century sits beside my reading chair, and most of what little I know about current European politics comes via him in some form or other.
Art’s official title is Senior Affiliate at the Center for European Studies at Harvard University. He’s been a professional translator since 1977, and he’s also a writer. As a professional translator, Art works with French and English, and has translated over 125 books in his career.
As an undergraduate at MIT in the 1960’s, Art worked on an early program for doing circuit design that was later used in an Apollo mission. He got his start coding on an 50’s-era IBM 650, and today he stays current with modern web apps. (He and I have had occasion to correspond about things like GraphQL and React.) So in addition to being a translator, he has legit OG geek cred.
What follows is an edited transcript of most of the first half of our conversation, which is available in its entirety on YouTube:
I started off by asking for Art’s general thoughts on the responsibility of the translator, and what he thought about the whole controversy around Amanda Gorman’s Dutch translation.
Art: The responsibility of the translator, in my view, is to represent faithfully what the author’s intention is. To do that, I don’t think that a translator needs to share an identity with the person being translated — however one wants to define “identity.”
In my career, I’ve translated more than 125 books from a range of disciplines, many of them in history, or the social sciences, or economics — you read the Piketty book, which was probably the most widely read of all the books I’ve translated.
Now, what qualifies me to translate in so many different disciplines? I’m not sure that anything does except intense study. Before I sit down to translate a book, I read widely in the field from which it’s being translated. Many, many years of experience have equipped me to translate in a variety of disciplines.
Of course, when you begin as a translator, you don’t have that experience, so you have to have some kind of affinity — some knowledge of the culture from which you’re translating.
That knowledge of the culture is acquired in various ways, perhaps by simply studying the language from books, perhaps by living in the country and having conversations with people there, by conversing with people who work in the disciplines, by reading widely from people in the disciplines in which you translate.
For a poem, like Amanda Gorman’s, the challenge is different. But simply being of the same race as Amanda Gorman is not necessarily the qualification that one needs. A black person growing up in France would not have the same experiences as a black person growing up in the United States. Black people in the United States come from a variety of different communities, not all of which share experiences.
So what are the relevant experiences for a translator? It’s very hard to say in advance... I think in each case, it has to be made case-by-case. Usually, the author has a say in the selection of the translator, but many authors don’t really have the tools to evaluate the qualifications of the translator.
To be frank, publishers don’t necessarily have the tools, either. But the advantage an editor has over an author is that editors who have worked with a number of translators in the past have seen a range of the work of a particular translator, and they’re perhaps better placed than the author ot make the choice.
But the choice is not easy, and I don’t think can be based on a simple definition of identity. What goes into a translation is so much more complex than what we usually mean by identity when we’re talking about political identities.
Jon: This is really useful to me, because one of the topics I have on my list is: can a machine really be a translator? This is something that Felix Salmon and I disagree on.
My take was, this is a translation aide, like a turbocharged dictionary. It’s a tool, it’s not an actual translator. A translator is a person. And my reasoning there was various, but part of it was: a machine can’t bear any moral responsibility for the translation.
You’re not gonna say, “the machine is sexist.” You can say maybe the programmers are.
I think the other piece of it, which you highlighted, is: if we’re gonna do a thing where the translator has to share a set of identity characteristics with the translatee, then a machine will never qualify in any respect.
Art: I think I agree with you entirely that a translator is a person and a machine is a tool that can be used by a person.
[Art describes how he uses MT as a translation aide when working in a non-professional capacity with one of the other languages that he knows but isn’t as proficient in as he is in French. Re-emphasizing the point that, for him, MT is a tool, and one that has come a long way since he first started using it.]
With respect to the Hungarian text you referred to that was criticized on Twitter for its sexism, I thought that the original text that was submitted was actually a trap laid for the machine. Because it gave a series of sentences that one might expect to elicit a sexist response from a machine tool. And it did as expected.
It had a series of sentences where, if you didn’t know the text and were simply translating based on cumulative historical experience of the language, which is what the ML translation tools are based on, you’d obviously come out with that sexist reading.
Now I didn’t know about the second example you brought up, where they used gendered pronouns, and the machine continued to be sexist despite having that reference. That’s the kind of mistake that a human would not make, but a machine would.
Jon: One of the philosophical issues here is that all these machine translation tools are trained on a corpus, so they embody the biases and prejudices and patterns that are in the corpus. And there’s no way for the machine, in the moment — a least not yet, although there is work being done on this — to steer the translation with reference to a style [sample] or a fact pattern.
So you can have this tool that embodies knowledge about a corpus of texts, but when it comes to doing the actual translation, you can’t steer it except in this very brute-force kind of way.
I think Google uses a set of rules to randomize pronouns in some cases. So there are things they can do that are complex, brittle, and rule-based. But it’s almost like spraying vinegar on spoiled meat — you’re just trying to give it a better odor or mask the fact that there’s something in the corpus that is not working.
But, to go back to your opener, you talked about how you read widely in the field. So as a translator, you’re coming to it with this body of knowledge of this corpus. But when it comes to a specific work, you’re trying to pull in the current context — from whatever area, be it sociology or economics, or whatever.
If I frame it in terms of responsibility, then, part of your responsibility as a translator is to represent the language in this area of specialization as it’s currently being used. Is that a fair way to phrase it?
Art: Yes, that’s right. But essentially, that’s what machine learning is also doing. The machine is trained on a corpus, and it’s trained on corpora in two languages — the source language, and the target language — at the same time.
That’s the same thing a professional translator has to do. When I translate history, I read history in French, and I also read history in English, in a similar subfield of history. Because you’re translating for a community. And the receiving community has its own conventions. And the conventions used by historians working in English are different than the conventions used by historians writing in French.
There are certain set phrases that are used to connote a certain relationship to a body of material, or a certain authoritative posture of the scholar writing. And those phrases, if you translated them literally from French, would seem totally out-of-place in English. What you have to find is an equivalent for the French phrase that establishes the same relationship of author to text that the French phrase does.
What the human does to establish that equivalence is also what the machine does, which is to read corpora taken from the same context.
The problem is that the engineers who set up the machine learning don’t have as clear a definition of these sub-communities that they’re translating from. Machine Learning might take a French corpus, like all of Wikipedia, and use English Wikipedia as the target text. That’s a convenient way of locating an equivalent body of text, but it doesn’t narrow down the communities as a human translator would.
A human translator would take a much narrower community — say, French historians, writing about the French Revolution, and look at texts by English-speaking historians writing about the French Revolution — to establish those equivalences.
I think that might be one direction machine learning engineers might want to go, to think in terms of communities, rather than a more universal corpus of texts.
Jon: Yeah, that’s a great point. Google Translate is one-size-fits-all, and this gets at why it feels to me like a dictionary and not a translator. Because there’s no implied community.
But I like this idea that a translation is from one community to another community.
I like your point about these idioms that — I guess in internet-speak we’d call them signaling — they’re tribal markers, it sounds like. You use a phrase in a certain way to signal a certain kind of status to the reader, and then you’ve got to map that into the same signal from a different community.
Art: Right. Exactly.
Jon: I agree with you about the Gorman situation, but then if I think about it in those [community-to-community] terms, I’m thinking: ok, if we’re going to frame this as community-to-community translation, then what if there’s a community on the other end of the Dutch translation that sees the Gorman poem as “theirs.” And they’re less concerned with fidelity, or with other kinds of classic translator things, and for them, the identity characteristics of the translator are part of the translation.
I don’t know, I’m being super charitable. I think a lot of this stuff is about food fights and signaling. But I’m trying to think charitably with this insight you just mentioned. And there are metalinguistic properties, like the color of the translator, that go into this community signaling.
This is only the first half of the interview. There’s a lot more up on YouTube, where we go a little deeper into more general translation issues. I stopped the transcription here, though, because we’ve now covered the main material that I’ve been subsequently thinking about as it applies to MT.
Some thoughts
Art said: “I think that might be one direction machine learning engineers might want to go, to think in terms of communities, rather than more universal corpora of texts.”
This would be ideal, but currently there are two practical barriers to this approach:
Training an LLM is expensive, so training one on a niche subfield is out of the question.
Even if cost weren’t an issue, you need a really large corpus for the model to capture enough of the language to make it sound fluent. And you probably can’t enough histories of the French Revolution in either language to make that work.
At some point, there will be a technical fix that more closely approximates the work that Art describes himself doing when he sets out to translate a text. Specifically, there are two components to Art’s method:
Have a lifetime of much exposure to both the source and target languages and cultures, via reading, speaking, and even living among native speakers.
Spend time doing focused reading in subcommunities that are related to the text you’re trying to translate, so that you pick up the particular idioms of those communities and can bring them over from the source to the target language.
Right now, MT is only doing step 1 — the wide reading of an entire corpus. There is currently no technical mechanism for doing the more focused reading in step 2.
This lack of a way to focus the model so that it translates in a way that’s more natural to a target community is behind most of the moral and political issues raised in the original tweet and in the dialogue, above.
Once we do have a mechanism for conditioning the output of an MT tool to fit a more community-specific reference, this will raise a whole host of new issues and disputes.
I have some recent Twitter threads that begin to explore these issues, and I may turn them into newsletter posts in the future:




As far as I'm concerned, Andrew Conner's example reveals a shortcoming of the translator a lot more basic than sexist bias or lack of nuance. The meaning of all pronouns should be entirely unambiguous thanks to the presence of a unique antedecent ("John", in the first sentence). Even if Google should somehow believe that "John" is a female name, the pronouns would all end up the same. My impression is that the previous, logical (as opposed to statistical) wave of AI would have gotten this one right. Apparently, whatever google is doing is unable to connect words that are more than 2 sentences apart. If this is a general limitation, then we can sleep easy, at least those of us with tech jobs beyond data entry...
> Right now, MT is only doing step 1 — the wide reading of an entire corpus. There is currently no technical mechanism for doing the more focused reading in step 2.
"Fine-tuning" models on a smaller, more focused dataset definitely exists in other areas of natural-language processing. I wonder why we haven't seen it applied to translation?