The horseshoe theory behind the MIT virus skeptic study
First they ignore you, then they... validate you?
I dearly wish I could say that this viral thread has misrepresented this new paper, and that a team of dataviz specialists and anthropologists from MIT has not just conferred a massive amount of legitimacy on a dangerous community of quacks and loons, but I cannot:

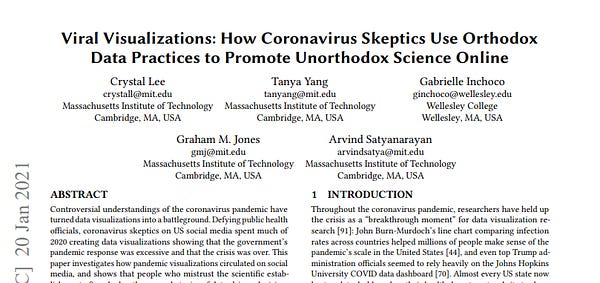
Note: In an appendix at the bottom of this article is a brief bit of background for readers who have come to this newsletter via my AI and tech coverage. Skip this if you followed my work on the pandemic throughout 2020.
If you want a summary of the paper, the Twitter thread above is pretty good. Basically, the paper argues that the skeptics:
Had a 👍 understanding of science as a process
Were duly skeptical of power structures and institutional narratives
Actively and effectively resisted establishment gatekeeping
Took time and care to educate one another
Were truly committed to the hard, brutal facts, shorn of sentiment and embellishment
Seem to have done some legit science, critically interrogated existing scientific hierarchies and gatekeeping practices, and made some 😍🔥 emoji dataviz. But also they were bad because some stuff about the January 6 insurrection, fake news, white supremacy, Big Tobacco, climate change denialism, and other culture war boilerplate, which we’ve crammed into this one section in order to send the correct signals to our readers.
I consider this paper and the larger dataviz project it’s a part of to be fundamentally misguided and even dangerous. Here’s why.
After ignoring these dangerous quacks, you come out with this?!
My first beef with this entire project is that it is the first serious scientific engagement I’ve seen from major academics with the likes of @EthicalSkeptic, Michael Levitt, Alex Berenson, and the other coronavirus cranks and skeptics, and that alone is an outrage. I begged on Twitter for the infectious disease community to take these folks seriously and to dedicate resources to debunking their work. Levitt himself routinely on Twitter and in videos begged the ID community to critically engage with his work. All to no avail.
Despite the fact that the Trump administration was clearly listening to these skeptic circles in setting public policy, nobody in infectious disease and public health — at least that I ever saw, and I paid close attention — was following skeptic twitter accounts, blogs, and Facebook groups, and engaging with them critically. The job of debunking all this stuff was let to journalists and non-specialists.
The systematic refusal to publicly engage with these bad actors was, in my opinion, a major institutional failure of science at all levels. The fact that this big project from MIT has now come out, and spends the vast majority of its text painting these bad actors in an extremely sympathetic light, is just the cherry on top of this crap sundae.
A massive data collection & visualization effort is a poor substitute for context and even-handed rigor
My second big problem with this piece is that the central figures in these circles — Berenson, @EthicalSketpic, and Levitt — have all made a number of predictions that have turned out to be wrong, and have routinely made claims that are clearly at variance with basic reality (more on this, below). Yet the paper doesn’t tell you how badly these big accounts misled their community, nor does it try to do any kind of post-mortem around how and why a community with this amount of apparent science awesomeness going on was so taken in by fantastic claims.
You could read most of this paper and the accompanying materials, and come away with the impression that these diligent citizen scientists were somehow productive, good-faith participants in our collective efforts to better understand the pandemic. Indeed, the paper seems keen on convincing you that what they were doing was in most substantial respects real science, and that in many cases they were doing it better than establishment scientists.
This paper’s authors have fallen into the same trap when assessing the virus skeptics that the virus skeptics themselves fell into when assessing the pandemic, specifically: data collection, tagging and labeling, filtering, and visualizations were substituted for detailed, domain-specific knowledge of the underlying reality that structured all these facts.
Let me give two examples, one from the virus skeptics, and one from the paper, to show what I mean.
Example 1: the flu-like fatality rate claims of the virus skeptics
Very early on in the outbreak, the virus skeptics started to claim that the fatality rate for SARS-CoV2 was roughly the same as that of seasonal flu. This claim has persisted despite all evidence to the contrary, and you can see it come up in recent tweets from Levitt and others.
There are three main ways the skeptics get to this point:
Rampant confusion of infection fatality rate (IFR) and case fatality rate (CFR). This is a pretty basic error I saw early on, but the more sophisticated skeptics are savvy to it and don’t tend to fall into it as much.
Claims that the real death count is overstated. This is the main tool for getting the fatality rate down into the ~0.1% territory where it needs to be in order to support a “flu-like” claim.
No understanding of or inquiry into how flu fatality rates are calculated, so that the comparison with COVID rates are apples-to-apples.
This last one — total ignorance of how flu cases are counted in the US, and how flu deaths are counted — was addressed early on by infectious disease experts, yet it persists to this day in virus skeptic circles.


Note that this actually gets at a second systemic problem with the work of the virus skeptics: their rigor and skepticism may have been real, but it was all entirely selective.
The COVID skeptics forged deep into the weeds of the material realities of data collection when it came to coronavirus numbers, but the flu numbers they were using as a standard for comparison went entirely unexamined. There just was zero curiosity about where those flu numbers came from.
Furthermore, the same lying US public health authorities and thoroughly compromised, opaque data collection practices that produced the questionable coronavirus data were suddenly infallible when it came to flu season and other seasonal illness data being used as a baseline. Again, the skepticism was quite narrowly focused.
TL;DR: The virus skeptics not only lacked critical domain-specific knowledge about where important numbers came from, but they were unwilling to learn it. They stayed focused on the parts of the data (and data collection practices) that interested them — and in this focus, they were quite sophisticated — but they didn’t want to look too hard at the provenance of any data that fit their just-the-flu narrative.
Example 2: the MIT paper focuses on the pretty charts, ignores the flimflammery
The anonymous Twitter account @EthicalSkeptic, who blocked me for criticizing his charts (he’s aggressive about blocking anyone who questions him), is one of the central figures in the virus skeptic network that the MIT paper identifies.
A lot of the paper is taken up with discussions of how legitimately great the virus skeptic charts were, and how expert this community was at wielding different data science concepts. Well, none was more chart-centric and data-science savvy than @EthicalSkeptic.
There was a reason this guy was one of the leaders in this effort — because he had the fanciest, most information-dense charts, and the coolest lingo. (Not only did he wow his audience with terms like “consilience” and “nose-over,” but he also made up his own specialized terms).
So instead of talking up how awesome the skeptics’ charts were, as in the MIT paper, let’s take a look at what the community’s main chart guy actually did with them.
The chart in the tweet below is very aesthetically pleasing, but you have to be something of an expert in @EthicalSkeptic’s data shenanigans to actually know what he’s taking an (undeserved) victory lap for. (Believe me, I tried to pin his followers down on what this chart was about and got no response other than being called a midwit.)
Using the Twitter advanced search tool, I went back and untangled the multi-month history of this chart on his feed (mostly by searching his timeline for “false lull.”, but also for “SARS”). What I found was remarkable: he claims to have called the end of the pandemic, to the day, back on 2/10/2020.

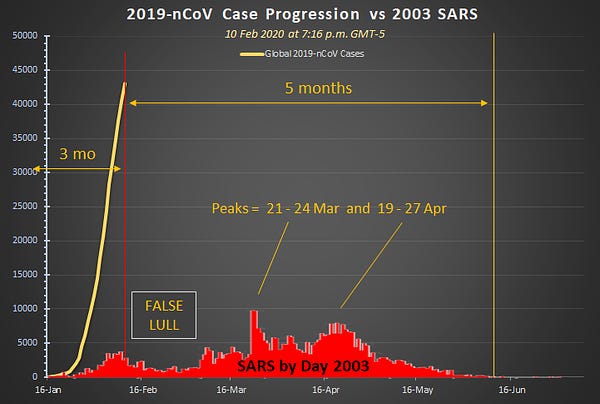
This tweet a few days later confirms that in the chart above he’s predicting a 6/16/2020 end to the pandemic:
To understand how he got here, you have to look at the original SARS outbreak curve from 2003 that runs along the bottom of his chart (in red in the above charts, and in gold in the later ones). That chart is a modified version of the one that appears on page 15 of this WHO document [PDF].
He not only believes that the SARS-CoV2 outbreak is seasonal exactly like SARS-1, but he thinks it follows the exact same epidemic curve — with the same peaks, troughs, and 5-month January-July timeline.

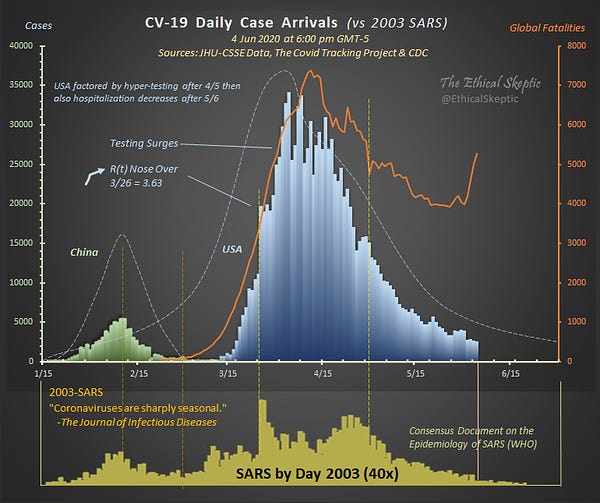

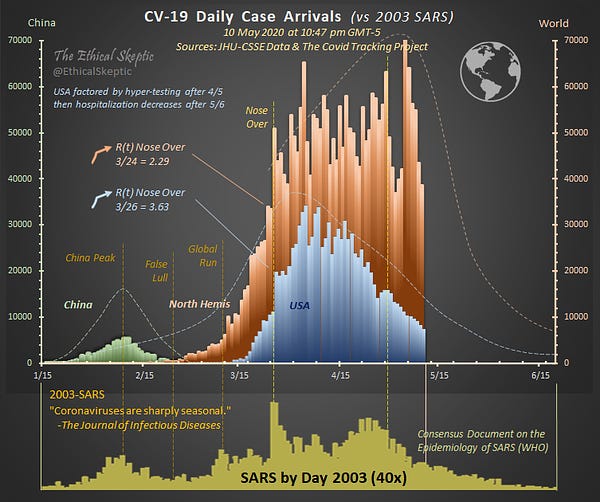
The idea that SARS-CoV-2’s outbreak was fated to follow the exact same curve as the 2003 SARS outbreak, and furthermore that its precise end date could be predicted months in advance, is bananapants. Just absolute crazytown. Yet @EthicalSkeptic took up the entirety of 2020 successfully peddling this insane idea on his Twitter feed using all the tools of data science that this MIT paper praises him and his fellow cargo cultists for.
And when his 6/16 deadline passed and the pandemic was still going, he dedicated his energies to “proving” that the pandemic had actually ended when he said it would, and all the case counts were just a bunch of fake numbers due to increased testing.
But you won’t learn about any of this chart abuse from either the MIT paper or accompanying website. The MIT authors went all-out with a bunch of data collection, AI-enabled classification and tagging, and data visualization, and spared not a single moment on the details of where anyone in the skeptic community actually went off the rails.
The MIT team is headed in a bad direction
I’m really struggling to understand the mindset behind this paper, or what agenda it’s intended to serve. I feel like there may be a hint of what’s going on in these two sections, but I really don’t like where this seems to be headed:
Similarly, these groups’ impulse to mitigate bias and increase transparency (often by dropping the use of data they see as “biased”) echoes the organizing ethos of computer science research that seeks to develop “technological solutions regarding potential bias” or “ground research on fairness, accountability, and transparency” [7]. In other words, these groups see themselves as engaging deeply within multiple aspects of the scientific process—interrogating the datasets, analysis, and conclusions—and still university researchers might dismiss them in leading journals as “scientifically illiterate” [74]...
For members of this social movement, counter-visualization and anti-masking are complementary aspects of resisting the tyranny of institutions that threaten to usurp individual liberties to think freely and act accordingly.
That footnote 7 in the quote above links to the ACM FAccT conference, the nerve center of “AI ethics” discourse that’s dedicated to criticizing scientific “gatekeeping,” along with “hierarchies,” “power,” “hegemony,” and so on.
I get a sense that the paper’s writers find something a quite admirable in the way that the virus skeptic community has positioned itself against the corrupt institutions, rigid hierarchies, and elitist gatekeeping of science, because this is exactly and precisely what the “fairness, accountability, transparency” critics of AI/ML and science in general are doing.
Indeed, if you replaced “anti-maskers” with “critical theorists” in the first sentence of the following quote from the paper, then it wouldn’t be out of place in much of the AI ethics literature I’m reading:
While academic science is traditionally a system for producing knowledge within a laboratory, validating it through peer review, and sharing results within subsidiary communities, anti-maskers reject this hierarchical social model. They espouse a vision of science that is radically egalitarian and individualist.
The place where the critical theorists — and seemingly the authors of the MIT paper — part company with the virus skeptics, is in the skeptics’ focus on individual rights and liberties.
In other words, these opposing cultural camps have a common enemy in modern science — corrupted by capitalism, hierarchically organized, wedded to gatekeeping, supportive of the status quo, etc. But the skeptics’ preferred alternative is more individuality, freedom, and individual liberty, whereas the the papers’ authors urge more “systemic” thinking and believe that a focus on individual responsibility can be “an ethics smokescreen to avoid talking about larger structural problems like white supremacy” (page 15).
I hope I’m wrong about all this, but this paper reads to me a bit like a set of left-populists examining a community of right-populists and finding a lot to like — if only the other guys would get off this individual freedom jag and become more collectivist in their thinking. As I often say on the bird site: horseshoe theory is real.
Appendix: some history on me vs. both the public health establisment and the virus skeptics
Here’s a February 6, 2020 snapshot of a page I authored containing detailed scenario modeling how the pandemic might play out in the US. If you scroll down, you’ll see that I warned in detail about everything from school closures to USPS slowdowns to supply chain disruptions and work-from-home — all of this a full month before Tom Hanks and his wife got suck with COVID and the whole world noticed the pandemic.
I continued to cover the pandemic throughout 2020, where I was early to flag the mode of transmission, and to predict different surges and the general contours of the catastrophe as it unfolded.
My here is not to take a victory lap, but to point out that I spent almost all of 2020 deep in the weeds of pandemic coverage, and I tangled repeatedly with both establishment scientists over everything from the likely severity of the pandemic (early on) to whether people should mask up (I said yes, they said no... before quietly pivoting to yes).
I also fought viciously and publicly with the virus skeptic community, and specifically with the accounts mentioned in this paper. I maintained a Twitter list of these people, and I did regular debunking of their lies and distortions.
The other bit of relevant background that some readers might not be aware of, is that before COVID I owed most of my Twitter following to my public advocacy for gun rights. This advocacy mainly involved educating followers about guns and gun laws, and also drilling down into “gun violence” studies, and bringing both math and basic gun literacy to bear on them.
I point this out, because like the virus skeptics I have spent considerable time publicly feuding with public health officials over an “epidemic.” And like them, I’ve done so by looking at the actual raw data and at the way the data was coded, and crying “foul!” when I found games being played with coding and/or conclusions.
So when the virus skeptic engine really got fired up, I recognized a lot of what they were doing with their “debunking,” because the angles of attack were ones I knew well from my time taking apart studies of mass shootings and gun violence.
But as I said in the sections above: details matter. The virus skeptics go off the rails because they refuse to learn basic facts about viruses and outbreaks, and gun violence researchers go off the rails when they refuse to learn basic facts about firearms and the gun market.
This paper is very irresponsible in implying that this virus skeptic community was "doing science". This is the very definition of Cargo Cult science and that should be made explicit. The paper should explicitly state how what they are doing is not science but simply using some science tools. In particular the virus skeptics could not put things in context, could not think critically, did not try to disprove their hypothesis, indulged in confirmation bias. All these need to be pointed out. I don't think the paper passed any sort of peer review?
The strangest part of that study to me was them equivocating anti-masking with being pro-school reopenings. That plus the Merriam-Webster definition of anti-vaxxer now including people who oppose mandatory vaccination has me feeling all sorts of label-confusion.