Stable Attribution: A New Tool Could Ruin Generative AI, Or It Could Save It
In this chapter of the story, I go beast mode on a newly launched tool
📖 The story so far: One subplot I’ve been following in the generative AI story is the artist backlash against Stable Diffusion. I haven’t yet dedicated an entire post to it even though it deserves one — instead, I’ve dropped references to it in previous posts.
This past Sunday, though, the launch of a new software tool called Stable Attribution represented a significant development in this particular story. So today is the day to take a closer look at how artists have responded to having their work used to train an AI that can then produce output in their style without their involvement, consent, or compensation.
What is to be done? Many people have thoughts and feelings, and some people are now writing software to “fix” the problem. With big changes, Stable Attribution might conceivably play a role in an eventual path forward. But for now, it’s an all-out war.
In a thread announcing a new service called Stable Attribution that claims to be able to take a Stable Diffusion-generated image and identify a set of “source images” in the generative model’s training data that gave rise to the generated image.

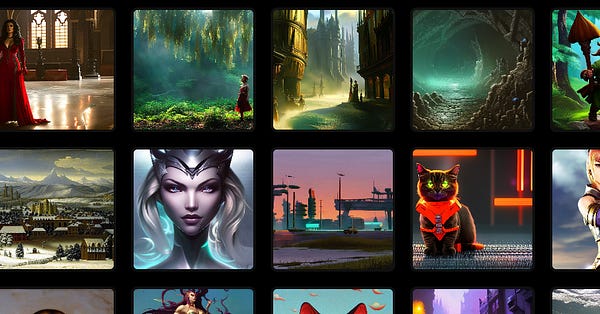
🤬 I immediately called BS in a couple of Twitter threads, which I’ll link below. I did not like Stable Attribution. At all. And it’s not because I hate independent creators and don’t want them to make any money. It’s because I am an independent creator and I don’t want to fight nuisance lawsuits.
This new site looked to me like a tool that profiteering lawyers will definitely use to send out mass shakedown notices to creators like myself, and/or that Big Content will use to kill decentralized AI. It also doesn’t do what it claims, either in theory or in practice (this is trivial to demonstrate). The whole thing is just an absolute fiasco, and I’m kinda terrified of it.
👼 But I think it can possibly be redeemed. The tools’ founders are good guys, and both their goals for the tool and their vision of how they imagine it being used are pretty benign and possibly even worth pursuing. It just needs a way different approach than the one they’ve taken; I’ll try to outline what I think can be done later in this update.
Some quick backstory on the AI art wars
💨 There’s so much to talk about just with this latest development that in order to bring you up to speed on the history of this story, I’ll have content myself with this very brief summary of events:
Stable Diffusion launches and goes viral after having been trained on the LAION-5 dataset, which contains many images scraped from Artstation and other online art venues.
Many artists are really, really mad about this because nobody asked them if an AI could be trained on their work. Also, the AI is shockingly good at producing new works in their style.
The artists protest in various ways. One of the protests was hilariously ineffective and premised on a complete misunderstanding of how ML actually works. (They thought that by replacing their art on Artstation with special protest art, Stable Diffusion users would suddenly get those protest images out of the model. I am not making this up.)
Online art services tried various ways to calm the situation down.
Stable Diffusion announces that Stable Diffusion 3 will include an artist opt-out function, where artists can go to a website and tell Stability that they don’t want their work used to train models.
There’s a lawsuit against Stable Diffusion which (falsely) paints the software as a digital “collage” tool and tries to make the case that every image output by SD is legally a derivative work of the images in the training dataset — this means if you use SD images commercially, you’d owe a lot of people a lot of money if the suit succeeds.
Stable Attribution launches with the shocking claim that if you upload an SD-generated image to it, then it can tell you which images in the dataset the model used to make that image.
So here we are the morning after the Stable Attribution launch, and after a Twitter Spaces that I participated in with the two founders behind the tool — Anton and Jeff.
💩 Here’s a summary of my initial, launch-day Twitter impressions of the tool’s core claim that it can take an SD image and do “attribution” where it identifies which images in the LAION dataset are somehow predecessors or sources of that image:
Demonstrably false, and probably nonsensical for most useful definitions of “attribution.” See this thread and the section below for more.
Potentially very useful to Big Content and very damaging to decentralized AI. See the same thread and the same section on problems below.
Or, potentially useful to decentralized AI and damaging to Big Content because the tool is so brittle and so unable to do what it says it may well function as a kind of live demonstration that the arguments in the anti-SD class action complaint are bogus. See this thread.
👍 I ended that Twitter spaces with a positive impression of the Stable Attribution creators and of what they imagine they’re trying to do, but they have seriously misunderstood the dynamics of content and I still think their tool in its present incarnation is bad bad bad.
The thing about artists…
Stable Attribution creators Anton and Jeff started out their journey with Stable Diffusion by tweeting valiant pushback against artists’ objections that the open-source tool was “stealing” their work. So far, so good.
But then they made a terrible mistake: they decided to listen to the artists.
👨🎨 Here are some hard truths about artists and indie creators and why it was a mistake for Stable Attribution’s creators to listen to them and then launch this tool the way it was launched yesterday:
Artists have no clue how any of this machine learning stuff works — this is especially true of the ones who are mad about Stable Diffusion. What little understanding the stridently anti-SD crowd does have is comically incorrect.
Artists have no leverage in our system of capitalism and intellectual property, and nobody outside their immediate family and a few fans cares about them, or their rights, or their earnings, or what they like and don’t like. Our entire IP system is built from the ground up to screw artists and creators and to exclusively benefit a small number of rent-seeking whales and tech-savvy law firms.
Number two is the really important one here because the Stable Attribution founders’ fundamental error was a failure to really understand the true power dynamics of the situation they inserted themselves into.
⚗️ What these two guys did was sort of like if a pair of well-meaning Medieval alchemists invented gunpowder, and then decided to set up a demonstration in the village square in full view of not only the peasants but also the local feudal lord’s knights with the pitch: “Oppressed people, we have invented a new weapon that will empower you to throw off the yoke of your master! So stand back and cover your ears…”
🏰 Every one of the peasants in the crowd would not just be watching the boom, but they’d be watching their lord’s knights watch the boom. Sure, some pitifully naive minority of the peasants might fall for the liberation pitch and get excited, but anyone with any sense would immediately grasp that the knights are about to take these two alchemists on a trip up to the castle and at some point soon the peasants and their families will have this powerful new weapon pointed at their faces.
The rational peasant reaction would be to mob these guys and end them and their invention before the knights can get their hands on it. That was definitely my reaction to Stable Attribution — “this can’t possibly be real because my mental model of generative AI doesn’t accommodate this, and to the extent that any judge or jury might think it’s real we creators need to kill it immediately before the lawyers get hold of it and come after us with it.”
😅 It turns out I overreacted a bit because Stable Attribution just doesn’t work as currently advertised and will never work as currently advertised. I’m going to end this post by insisting they advertise it differently, in a way that might actually turn it into a force for good, but first let’s talk about why it doesn’t work the way they’re claiming it does.
How Stable Attribution (doesn’t) work
Per some discussion of this tool on Twitter and in Anton’s own conversation in last night’s Twitter Spaces, Stable Attribution works roughly like this:
A user uploads an image that was generated by Stable Diffusion.
The tool uses its knowledge of the weights in the SD model file to augment and improve what is essentially a similarity search of the training data, i.e., “retrieve the images from the training dataset that are most like this input image.”
The tool displays a set of images, ordered by a similarity score, that it tells the user were the “source images” that were “used” by Stable Diffusion to generate the uploaded image.
To see it in action, check out this screenshot of the site’s results after I uploaded a Sasquatch that I generated via Stable Diffusion for an earlier article. You can click here to see the page this is a picture of.
The technical details for how they came up with that list of “source images” are scarce, other than what’s in this recent thread:


👯♀️ Their method is essentially a similarity search but heavily augmented and improved with a bunch of work that his company uses for ML explainability. It seems like they’re using the model weights file to help steer the similarity search in such a way that you can plausibly tell a math-based story that goes something like, “these are the training images that are most likely to have influenced the model’s generation of this output image.

He says in the above tweet, “it’s not wrong to say that…” It’s also not necessarily right.
I think calling what’s going on here “attribution” — as in, we can positively attribute these SD output images to these predecessor “source images” in the training data — is an enormous stretch of the concept of attribution. I am not alone in this estimation.

Actually, I think it’s way more than a stretch — I think it’s dangerously irresponsible and they should stop using the word “attribution” immediately. I’ll explain why in a bit.
🧚♀️ All of that said, I imagine there is certainly a story one can tell at some level of abstraction that will sound like “attribution,” and I further imagine that this team could conceivably improve on both the tech and the story to a point where a jury may swallow it. This will require a certain set of metaphysical commitments about “authorship” (machine vs. human) and a willingness to follow along with something like the following chain of reasoning:
If Stable Diffusion had not been trained on these similar images we’ve identified in the training data, then it probably would not have produced the image you just uploaded (but we can’t know that for certain, because with the right prompt and the right seed any output is theoretically possible, though outputs that are very distant from the training data are unlikely enough to be practically impossible).
Ergo, these images we’ve identified in the training data are the “source images” for the image you just uploaded. The model “used” those images to make your image.
QED
🚮 I want to be clear: as an argument about attribution and who gets credit for what, the above is flimsy and very hard to credit. At its core, it relies on a counterfactual combined with multiple probability estimates (the similarity scores of the images plus the relative odds that you’d have generated that image had the high-scoring images not been in the training data). This is all nerd fantasy stuff that will not hold up in any court, nor should it.
Important note: There’s a chance I’m wrong with the above and there’s a totally different logic implicit in the team’s reasoning about attribution. If I become convinced that this is the case, I’ll update this post.
🛑 But the biggest problem with this tool — at least in its launch iteration — is that by its own standards, it simply doesn’t work.
Check out this picture of some Ewoks done in an impressionist style. Stable Attribution “attributes” this image to a whole bunch of Disney-owned assets that will make Disney and their lawyers extremely happy, but there are zero impressionist painters among the results. Not a one.
I will concede that this is the kind of thing they can probably fix with further tweaks to their similarity search. I imagine a future version would dig up some impressionists to go along with the Disney-owned IP it founding the training data.
🚨 The problem they cannot fix, however, is that the tool has no way of determining if an uploaded image was generated by Stable Diffusion or not. This is a fundamental problem that the developer admitted in the Spaces chat may not even be theoretically possible to solve.
So right now and probably until the end of time, if you present this tool with a human-made image, it will happily point you to a set of “source images” in the SD training data that it falsely claims are behind the image you gave it. Take a look at what it did with this Soviet propaganda poster I uploaded:
The claims on that page above are just 100% false. There’s no sugar-coating it.
Now, Anton the developer has repeatedly insisted that uploading human-made images will of course break the product in the manner I’ve demonstrated here. He thinks this is a warning about misuse, but it’s really not — it’s an admission that Stable Attribution does not and cannot work as a technology for “attribution.”
Again, there’s really no fix for this, and if you scroll back up and work through the chain of reasoning I laid out behind the “attribution” claims, you can see why there’s no fix.
🔑 My own intuition is that the fundamental inability of this tool (or any other hypothetical tool) to definitively determine if a given image was or was not created by Stable Diffusion is the key to destroying the anti-SD class action complaint’s argument that all SD-generated images are necessarily derivative works of the training data. I think there’s a big unlock in here, and I also think the Stable Attribution team should drop all this “attribution” language and pivot to trying to find that unlock.
What the team was hoping to achieve
🎈At some point in the Twitter Spaces, I floated the following scenario, and I floated it because I already suspected it was the desired end-state the team was hoping for when they launched Stable Attribution. (They confirmed that it was.)
Instead of whatever tiny fraction of a penny PlaygroundAI charges users for a generation, they instead charge, say, twice that fraction.
For each generation, Playground would use Stable Attribution to get a list of the training data images that are most similar to the generated image.
Playground would then pay out half the money the user paid the platform for that generation to the rightsholders for the images identified by Stable Attribution.
If this were to happen, then artists whose work is included in the dataset would be getting a small micropayment every time their image is used in a generation. The more popular their art style with Playground users, the more money they’d make.
And in this hypothetical world, artists would want to have their work included in Stable Diffusion’s training data because this would give them a brand new revenue stream for their work.
💸 In the Stable Attribution founders’ vision, platforms would opt into this arrangement willingly, because it lets the artists get paid. And when the artists get paid, they stop protesting decentralized AI image generation tools and start cheering it on and supporting it by contributing their own work to it. Everyone’s incentives would finally be aligned, and everyone would be happy — the artists, the platforms, the users, Stability AI, and content creators like me who are using these tools for work.
🙌 This all sounds wonderful. I say that unironically. This would be great if they could pull it off. But there are big problems.
🤦♂️ First, to the goal of satisfying artists so that they stop fighting Stable Diffusion — this was a bad goal and they should not have pursued it. As I said above, indie artists truly are not a threat to decentralized AI, because they have no leverage of any kind in our system. They have no money and no real source of influence, and their only possible role in this entire drama is as patsies for Big Content.

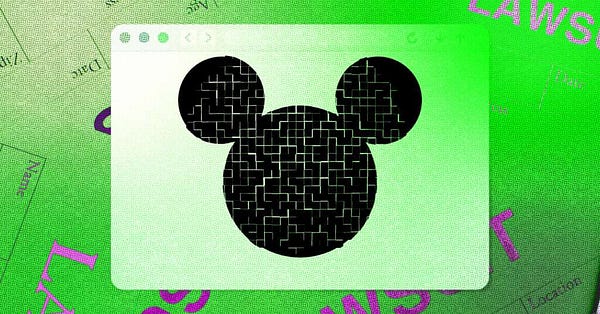
I realize I’m speaking harshly, but these are just facts. We live in the world of the Golden Rule, which is, “he who has the gold, makes the rules.” The artists have no gold and make no rules. They have no real agency and are not a direct threat to any party in the AI wars. They’re pawns, not players.
Disney, on the other hand, has tons of gold, so it makes all the rules of the current content game. Disney is the player, so when anyone sets out to work on attribution and explainability as it relates to IP and the flow of payments, their first and foremost thought should be, “how is Disney going to use this to screw creators?” That is the threat you work to mitigate — not the artist protests, which, again, do not matter.
This brings me to why I am worried about this tool.
Why I’m terrified of Stable Attribution
🥚 As a creator and a publisher, I have to be very careful about “feature” or “hero” images in my articles— the big image at the top of an article that shows up in the promo cards on Twitter and in other places.
🏴☠️ There are law firms that specialize in suing people like me for using some creator’s copyrighted image in an article. They use automated web scraping and other software to send shakedown letters to creators demanding thousands of dollars per alleged infraction.
These automated legal shakedown campaigns are also very commonly carried out against YouTubers and Twitch streamers based on images and audio that show up in their streams. All of this is a huge hassle to deal with, even if the claims are bogus. And every now and then, a claim isn’t bogus and you have to pay up.
Law firms aggressively recruit photographers and other indie artists to “retain” them by promising them a revenue stream from these shakedown campaigns. This is presented as artists just getting their due, but the lawyers take a big cut and make tons of money by rolling up lots of these rightsholders so that they can run their automated campaigns at scale — just printing money by scraping the web and mailing legal demand letters.
This terrible situation has a few important implications for how I and other creators present our content online.
1️⃣ First, unless you’re dumb or naive, you only use feature images from a handful of websites that are safe because of the strict licensing rules they enforce. (My current list is Pixabay, Pexels, and Unsplash.) You do not use Flickr, even filtering by CC licenses, because you can still get nailed.
2️⃣ Second, some streamers and other creators are often forced to pull content offline rather than hassle with the takedown notices.
➡️ The end result is that everyone lives in constant fear of legal shakedowns, which is why so many of us rejoiced when Stable Diffusion was launched. Here was a source of unlimited, novel feature images that were by default totally unencumbered by others’ IP claims.
(Sure, if you use SD to reproduce a copyrighted image from its training data, which can happen, and you commercially exploit that image then you’re still in trouble. But that has nothing to do with SD — you’re in trouble because you’re commercially exploiting someone else’s copyrighted image, and it doesn’t matter if you got from SD or you right-clicked and saved it from the web.)
☠️ What this meant for me, is that when I looked at Stable Attribution all I could see was another tool in the automated legal shakedown toolbox. I’m pretty sure I was not alone in that reaction, either. This is why I had (and still have) a viscerally negative reaction to this tool. I can guarantee that if it continues to exist in its present form, lawyers and Big IP will use it to screw me and other creators.
💣 If Stable Attribution stays online and anyone successfully gets a court to recognize it as a valid method of identifying source images for derivative works, then I will ultimately have to remove every SD-generated hero image on this site or risk getting a string of automated shakedown letters from law firms that specializes in representing rightsholders whose work is in the LAION dataset.
How to fix this fiasco
It may be possible to turn Stable Attribution around and pivot it into the kind of tool that can be used to realize its creators’ original vision — users pay services like Playground, Playground uses a similarity search on each generation to find related images from the model’s training data, then artists get a cut of the revenue and are incentivized to include their work in training datasets.
But to get there while also avoiding the world I’m worried about — the one where I’m getting shakedown letters from profiteering lawyers or from Disney for every SD generation in my newsletter — is going to take a major change of tactics.
💭 Here are the steps I think would work:
☄️ First, rename the app immediately, and scrub it any and all language about “attribution,” “source images,” and which images the model “used” to create which other images. Kill all of that with fire, because it’s not necessary, it’s not accurate, and it’s going to get us all sued.
🎮 Next, reframe the entire process as a kind of multiplayer game with a similarity search at its heart. The game works like this:
Players contribute images for training
Model makers like Stability AI train their models on the images and publish the results for online platforms to Playground to use.
Platforms run the models for paying users, running a similarity search that produces the N most similar images from the training dataset.
Platforms pay the winning rightsholders whose images were in that N-sized list of similar training data images.
👉 There is no “attribution” here. There is no “source image” or “derivative work.” It’s strictly, “Hey there, creator, you just won this lookalike contest! Our algorithm scored this user’s output image as similar to an image you own that you entered into the contest, so you win money!”
(Ok I’m not really wedded to the “game” part, but you get the idea. It’s an arrangement of some sort that explicitly and deliberately has absolutely nothing whatsoever to do with attribution. It’s just about similarity scores, which are not indicators of anything other than superficial similarity.)
👨💻 Ideally, investors in AI and other stakeholders would set up and generously fund a non-profit foundation to create and operate a rightsholder portal where rightsholders can create accounts, contribute images to this game, and get paid for winning it.
✍️ In order to sign up for payment, rightsholders should have to sign a kind of anti-licensing agreement — a statement or affidavit that testifies to their understanding of the following key terms and realities:
The rightsholder is not licensing the work to the model maker, because such licensing is not necessary for training models.
The resulting model file will not be a derivative work of any of the training images the rightsholder is contributing.
The images that are generated from the resulting model files are not inherently and automatically derivative works of any of the training images the rightsholder is contributing. Sure, the model may produce works that are similar enough to the training data to be derivative works — that’s always possible. But there’s nothing inherent to the process of training and inference that establishes a “derivative work” relationship between the training images and any output image.
Once they’ve agreed to the above, then they use the portal to contribute images either by uploading them or identifying them in a dataset and proving they have rights to them.
💰 Rightsholders are paid up-front some per-image fee with a maximum cap — so let’s say $10 per image for up to $1,000 per rightsholder for contributing their images.
So the flow is:
Log into the rightsholder portal.
Sign the affidavit or statement.
Enter bank account details for payment.
Upload or tag images.
Get paid up-front for up to $1,000.
Sit back and collect more payments if your images are popular on participating image generation services.
If you imagine 1 million rightsholders collecting $1,000, that’s only $1 billion — very easily within the investment community’s ability to come up with for the purpose of funding a foundation or non-profit that could end the threat that Big IP poses to generative AI and secure an endless stream of training data where artists get a share in the upside.
🫡 If this were to happen as described, then AI companies like Stability AI would have a huge war chest of signed understandings from rightsholders to the effect that they all understand that generative AI is not stealing or otherwise misappropriating their work, there is no licensing necessary to train models, and there is no inherent connection beyond superficial similarity between a given training image and a given output image.
🍻 Artists, for their part, would have a brand new income stream from their art, and all the other benefits described in the section on what the Stable Attribution team was hoping to achieve.
🤕 As for Disney and the profiteering shakedown lawyers, they would be facing a legion of creators who are all willing to testify that their rights are not being infringed because generative AI does not work by remixing source images into derivative works.
Postscript: the moral angle
❓Backing up briefly to my Sasquatch picture, I want to bring up a question that I asked of the Stable Attribution creators in the Twitter Spaces event last night. I pointed them to the Sasquatch page on their website, asked them to look at my picture on the right and at all the pictures on the left that their tool had identified as “source images,” and tell me why they believe I owe the owners of any of those images any money.
I never got an answer for this.
🙊 Leaving all the legalities and technical realities aside, nobody in the Spaces event was able to look at that link and make the moral argument that my commercial use of my Sasquatch image has indebted me in any way to the creators of the images alleged by Stable Attribution to be its “source images.”
🤡 I think they could not do this because the idea is ridiculous.
If you look at my image and the supposed “source images,” they’re clearly very different and only superficially similar in some vague, stylistic way. If my Sasquatch had been made in, say, Photoshop, no judge or jury would compare it with those other images and rule that I owed their creators any sort of credit or attribution. I think no artist would look at those images and decide I owed anyone credit for the Sasquatch. It is absurd on its face.
So I think the attribution language on this website, in addition to being wrong and dangerous, is just extremely silly. LOL absolutely not, guys. You are yanking my chain with this. I dislike having my chain yanked. That is my freaking Sasquatch and if somebody comes after me over it waving a page of your similarity search results then we got business. 👺
On the other hand, if it’s clear to all involved that the only thing happening is mere similarity search, and PlaygroundAI wants to pay the owners of the top N results some micropayments because they came up on some internal list when I generated that Sasquatch with the service, then I don’t care. Whatever. As long as the upcharge isn’t enough to send me looking for a cheaper service, it’s none of my business.
🏆 Removing this farcical “attribution” language changes the entire character of the exercise from a mortal threat to creators like me into a fun pattern-matching game where everybody wins.
As a small time art hobbyist with a nonzero but very small amount of technical background, the AI art wars have been a fascinating, if tense, story with major implications for how people like me can navigate the waters of creating content and putting it out there in an increasingly changed market and environment.
Unfortunately, most of the level of discourse that runs in my circles is between Team Artists (i.e. people who have apparently never taken a single computer or copyright law-related course in their lives) and Team AI (a group of teenagers who believe at least 90% of computing power in existence should be redirected towards the sole goal of generating images of clinical macromastia in anime style).
I can't speak for anyone else, but your work on this subject has been eye-opening without the editorializing or bullshit of either side. I don't know how much work you put into researching and writing these posts but I can say that for myself and others like me not infected by internet brainworms but who can't find an unbiased source to learn about all this stuff, the entire post dedicated to the AI art wars would be greatly appreciated.
Cheers and best wishes.
I 100%agree. thanks for writing this.