Should the law require Alexa to speak like a social justice activist?
"I'm sorry, Dave. I'm afraid I can't say that."
The Financial Times has posted a new editorial that drafts on the recent departure of AI ethicist Margaret Mitchell from Google, to warn of the dangers of large, powerful AIs built on "unrepresentative" datasets that reflect "historic biases."
To address this problem, the piece insists that three rules must apply to the development and use of AI:
"Teams that develop AI systems must be as diverse as possible to reduce the risk of bias."
"Complex AI systems should never be deployed in any field unless they offer a demonstrable improvement on what already exists."
"Algorithms that companies and governments deploy in sensitive areas such as healthcare, education, policing, justice and workplace monitoring should be subject to audit and comprehension by outside experts."
This stuff is a long way from Asimov's Three Laws of Robotics, but what both have in common (apart from the rule of three) is a focus on harm. Oh, and also the fact that they're meant to be laws — not optional recommendations. The FT's authors point to a specific piece of legislation, H.R.2231 - Algorithmic Accountability Act of 2019, as a first step in implementing this three-part agenda. They'd like to see a lot more such steps.
Unrepresentative of who?
The FT editorial opens with a central yet false claim that goes directly to an issue at the heart of the entire AI ethics debate: what does it mean for a set of training data to be “unrepresentative”?
The FT authors summarize Mitchell and her coauthors on the "Stochastic Parrots" paper as arguing that the large language models Google and other companies employ "rely on unrepresentative data sets. [emphasis mine]" But this is just not true, and at least in the "Stochastic Parrots" paper this term is not used.
The problem Mitchell, Gebru, and their allies repeatedly identify with AI training data — not just in this latest paper but more generally across the AI ethics' fields years of work — is precisely the opposite: these datasets are too representative of our actually existing (problematic, colonized, cisheteropatri-all-the-things) world.
The AI ethics activists don't like that these large AIs are trained on data from the normie world of microaggressions, gendered language, and implicit biases — they're trying to destroy that world, so they'd not see it perpetuated by a globe-spanning, energy-sucking artificial intelligence.
One might say that in the taxonomy of critical race theorists described by Richard Delgado and Jean Stefancic in Critical Race Theory: An Introduction, the AI ethicists who'd sanitize the world's problematic datasets seem to be "idealists":
One camp, which we may call "idealists," holds that racism and discrimination are matters of thinking, mental organization, attitude, and discourse. Race is a social construction, not a biological reality, they reason. Hence we may unmake it and deprive it of much of its sting by changing the system of images, words, attitudes, unconscious feelings, scripts, and social teachings by which we convey to one another that certain people are less intelligent, reliable, hardworking, virtuous, and American than others.1
To paint a more concrete picture of what this might look like: the ethics people want to be able to do for the language used by consumer products like Alexa and the Google search box what activist orgs are already doing to great effect for the media, i.e., circulate a guide for what to say and what not to say (like this, or this), so that we end up with headlines that have X's sprinkled through them where vowels should be, or with constructions like "vulva owners" instead of "women."
One gets the sense, fairly or unfairly, that they'd like Google to tell you, "I can't find any results for 'breastfeeding.' Did you mean, chestfeeding?"
As a society, we're now facing this same issue everywhere, including in the innards of these AI models:
Will we permit the continued existence of large, public representations of "problematic" worldviews — children's books, TV show episodes, school names, statues, machine learning models trained on normie vocabulary — or will we purge all this stuff and reset everything in our culture to fit with new, woke norms?
There are only two possible answers to this question: “yes” or “no.” You may be allergic to binaries, but you will nonetheless have to pick a side on this one, eventually. It is not going away.
Will researcher diversity make the algos more just?
The first of the FT's proposed rules ("Teams that develop AI systems must be as diverse as possible to reduce the risk of bias.") corresponds to an avenue I wanted to go down in my Google Colosseum post, but couldn't. Why? Because it's a really short avenue that quickly leads to a dead-end.
To be specific: I have yet to see a single argument laying out in any detail how bumping up the skin-tone hex code on the AI brain trust — by "brain trust" I mean the handful of highly paid uber-nerds who develop the algorithms that implementation engineers then train and release into the world — will lead to social justice changes in commercial machine learning models.
Indeed, there are two things here that are routinely hand-waved over in these discussions:
How the mathematics, or even the selection of certain functions and benchmarks, would be different if the whiteboard crowd were less white.
The fact that a non-trivial number of the researchers in any given company's brain trust are Asian or Jewish. (At least, this is so in my experience and that of many ML people I’ve talked to.)
I'm going to go ahead and propose that this stuff is hand-waved over because it is not real. And it is not real, because incentives trump identity.
Any group of black and brown math nerds, given the same market pressures and business mandates as the Asians, Jews, and white guys that make up the AI teams at companies like Google and Facebook, will produce work with biases that are indistinguishable from what we're already seeing.
Change my mind.
The heckler's veto
The FT's second rule is a doozy: "Complex AI systems should never be deployed in any field unless they offer a demonstrable improvement on what already exists."
They are very straightforwardly and openly asking for a veto on the market's ability to meet demand with products backed by machine learning.
I see this specific demand — that ML workers should first question if a product should even be built, then maybe don't build it — come up again and again and again from certain quarters.
Here's a random example that came across my feed just yesterday:


And here's a short thread from me on an entire paper I came across this week arguing that a whole category of ML tasks probably should not exist:

This same argument — maybe don't ever build a machine learning model that tries to infer gender from text or images, because the very idea is problematic — comes up in a widely circulated talk by Timnit Gebru:
You might imagine that this veto power over what kinds of ML models should exist and what kinds should not, is to be ultimately vested in a democratically elected government. But so far, you'd be mostly wrong.
Congress moves too slow for language that changes at the speed of woke, so the movement needs a way to get out ahead of problematic ML before it even gets deployed. So if you follow the activism in this space, you quickly realize that DC isn't where the main fight is.
Instead of government regulation (or maybe, in addition to regulation), the AI ethics activists would like to see AI researchers and engineers themselves deciding that this or that problem should not be solved by ML because it might cause "harm."
This bottom-up veto idea is behind a lot of the tech workplace organizing that's happening right now. The veto power is to be vested in the Google union, where the activists can work from the inside to ensure that certain subjects are not even broached, certain tasks never proposed, certain categories of problems never even considered.
It's also behind the unending Twitter pile-ons, where researchers are publicly browbeaten into retracting conference papers because of problematic language. For instance, this researcher's paper assumed a gender binary, which enraged a non-binary colleague.

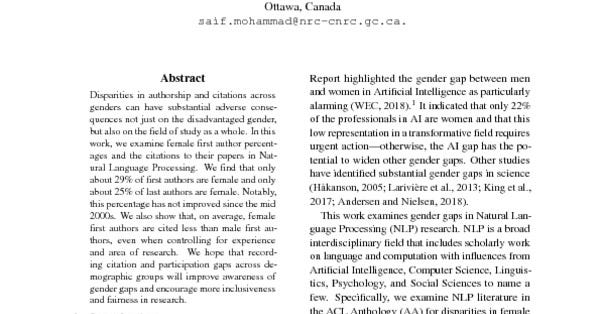
The offending researcher quickly caved and tried to retract the paper:

These pile-ons (and cavings and retractions) are happening routinely, not just on Twitter but at conferences. The ultimate goal is to hand over control of the research agenda for the entire AI/ML field to a loud, aggressive minority that would set itself up with veto power over what questions can be even be asked.
I do not actually think they would disagree with my characterization, here. Like Ibrahim X. Kendi’s idea of a kind of all-powerful anti-racism committee that gets the final word on whether any part of our society is racist or not, and has the power to reorganize it if it is, the AI ethicists are not shy about proposing that they be in charge of absolutely everything.
Clearly, they’re going to have to kill capitalism before they seize the market’s deciding power for themselves. That bigger project is well underway, I guess.
An algorithmic FDA
The third proposed rule is one I'm actually somewhat sympathetic to, but it’s also the one that worries me the most: “Algorithms that companies and governments deploy in sensitive areas such as healthcare, education, policing, justice and workplace monitoring should be subject to audit and comprehension by outside experts.”
Those who follow me on Twitter know I'm an anti-monopoly, pro-regulation capitalist. I unabashedly believe a democratically elected government has an active role to play in mitigating many of the downsides of capitalism. But I part company with many on the left when it comes time to actually define and measure capitalism’s downsides, or “harms.”
So if the proposed regulation looks anything like a Kendi-style algorithmic justice committee, where appointed bureaucrats and activists are deciding which models are harmful and why, I’m going to be on the opposite side of that fight, sharing a foxhole with the free market folks.
However this shakes out, though, I can heartily endorse more transparency and better auditing tools at a minimum. Whatever we do by way of regulation will depend on how good we are at measuring the real-world impacts of ML systems — both before and after deployment.
We do this kind of measurement and regulation with power systems, financial systems, and other critical technologies, so my instinct is that we can do it with ML. Indeed, I think we will probably have to. But I'll develop that idea more in a later post.
As for the new proposed law, H.R.2231, it is actually not that directly connected to any of the stuff the FT editorial is arguing for — it's mainly about privacy and personal information, and how companies store and use that information. So it's a kind of camel's-nose-under-the-tent effort and not a big step.
Addendum: housekeeping
Yoav Goldberg posted a response to "Stochastic Parrots" that covers some of the same ground as my previous post, Google's Colosseum: A criticism of "On the Dangers of Stochastic Parrots: Can Language Models be Too Big". I was almost done with my own draft when I came across this, but I do find it useful and may write about it more, later.
This piece on The Gradient, Lessons from the PULSE Model and Discussion, by Andrey Kurenkov, is really the definitive roundup of Tweets related to the original LeCun vs. Gebru argument. I don't know what I think about Kurenkov's attempt to draw actionable lessons from this mess, but I think he did amazing work in gathering all this material together and organizing it. My Colosseum piece benefitted from it, greatly.
Critical Race Theory: An Introduction, Delgado and Stefancic. Page 21.