Issues in AI: moral responsibility, skepticism, and more regulation
This update has zombies in it
Here’s a brief Friday link roundup of some things I’m reading and thinking about. As always, if you have thoughts or pointers to further info, please drop them in the comments. I’m not always able to respond, but I’m trying to dedicate time to being more active in the comments section.
AIs and moral responsibility
There’s an emerging discourse I’m tracking that goes something like the following:
AIs actually do not have explainability problems, and the whole opacity/black-box discourse is just deployed as a way for AI’s makers/sellers to avoid taking responsibility for the things their models do.
Because AIs aren’t any more mysterious or opaque than, say, human institutions, we can certainly reason about them and regulate them the same way we do human institutions.
Because we can easily reason about them, make predictions about them, and regulate them, therefore they will always have the moral status of mere tools and can never be responsible moral agents in their own right.
The point of the above is to frame all suggestions that AI is opaque and hard to regulate as Big Tech attempts to avoid regulation and responsibility. Practitioners who object to this will be alleged to be compromised because they (almost always) work for Big Tech, so their interest is in portraying AI as something new, mysterious, and difficult to control.
Anyone who has spent any time learning about financial regulation will recognize the above dynamic, where informed insiders protest that it’s complicated, and would-be regulators from outside disagree and accuse the insiders of trying to hide malfeasance and greed behind a veneer of phony complexity.
Indeed, I most recently saw this come up in a machine translation debate, where finance journalist Felix Salmon deployed a version of it against Google Translate’s problems:

My initial reaction to this type of thinking, when applied to AI, is extreme suspicion. The work I’m reading in this area seems so far to be a bunch of motivated reasoning aimed at making various policy and regulatory cases, and the claims often just don’t square with what I’m learning from actual ML practitioners.
But I’m not going to spend time yet critiquing any of this in detail, because I’m just now starting to get my head around it. I also reserve the right to change my mind about it as I read more.
I do want to flag some examples of this work, though, because I can see the appeal of the story it’s telling and I suspect this discourse will develop into something I’ll see a lot more of in the coming months and years:
Again, linking is not an endorsement. I’m not a fan of the stuff above, and if the kind of thinking represented in it catches on then I’ll take a crack at explaining why I don’t like it.
In lieu of further analysis, I leave you with a thought experiment:
You go to the doctor with mysterious symptoms, and the doctor sends you out to a lab to run a bunch of tests. The lab uses a brand new medical AI to evaluate the results and give a recommendation to the doctor.
The AI says you need major surgery or you will die soon. The doctor says this is nonsense and does not at all square with his own diagnosis. So you get a second and a third opinion, both of which concur with the doctor against the AI’s recommendation.
Now, really imagine with me that it’s your life on the line here. Don’t you want to know how the AI arrived at this conclusion? What its reasoning is? The doctors can give a verbal account of their reasoning, so won’t you insist on something similar from the AI?
Keep this scenario in mind, and then read this article and see if you agree with the author’s dismissal of the demand for more fine-grained explanations of how an AI arrived at a particular decision.
A good reminder for AI skepticism
There’s a ton of money flowing into AI right now, and as a consequence, there’s a lot of snake oil being passed off as high-tech. This annotated slide deck is a good overview of the current state of play in what’s AI snake oil and what isn’t.

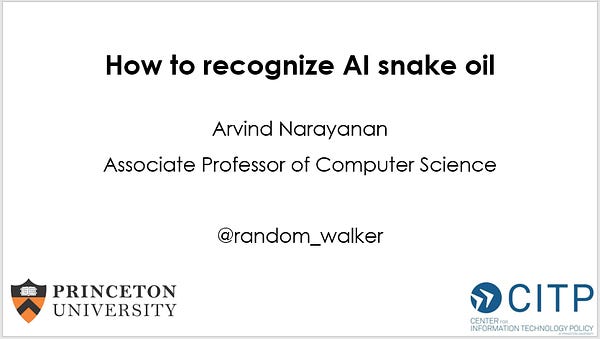
I want to skip straight to page 10, which has a good summary box of the contents of the talk:
This certainly captures the state of things right now, but I think this talk wants to make a move that I see a lot of in ethical AI discourse: jump from “AI can’t currently do it, and shouldn’t even be trying” to “it can’t be done with AI.”
The author is aware that this move amounts to a hypothesis, and indeed he gives this as his key falsifiable claim: “For predicting social outcomes, AI is not substantially better than manual scoring using just a few features.”
This claim may turn out to be true, but I think we should all plan for the possibility that it turns out to be quite spectacularly false.
We should be asking ourselves: what would society look like if ML could do substantially better than any other technique at predicting social outcomes in the areas listed in that box?
It’s better to think through this now while it is not true than to wrestle to it after it is not only true but deployed in production somewhere.
Big tech and regulation
There are some more reactions going around to the EU AI regs I mentioned the other day, but one interesting wrinkle I wasn’t aware of was the fact that Google is actually pushing for regulation and is supportive of the draft’s sequestration of some types of AI into “high-risk” categories.
I learned of this recently via a video of an October 2020 panel on EU AI rules, wherein the Google talking-head lays out the search giant’s position on the new rules.
I have to confess that my reaction on hearing his segment, was that of course he’s for it — as I said in yesterday’s update, it’s the kind of regulatory thicket that only a BigCo can ever hope to navigate.
As for the aforementioned reactions, the only one I’ve seen so far that I want to highlight is this argument that the draft doesn’t go nearly far enough in terms of labor protections from the US of AI in the workplace.
I think we’ll see a similar argument replicated in a lot of areas — it doesn’t go far enough. I can already predict that the fact that it doesn’t really tackle a number of task categories AI ethics folks have identified as inherently problematic (i.e., race/gender/sexuality inference) will be raised as an objection.
Finally, stateside Google and other big tech platforms have their fingers in various states’ platform privacy laws. The Markup has an overview that looks good, but I haven’t been following this issue closely so I don’t know how good it actually is. If you have better links on this topic, please drop them in the comments.