EU set to kill AI startups; how chip shortages are like gun shortages; Twitter's unsolvable AI ethics paradox
Also included: more developments & thoughts on the TSMC and Taiwan invasion issue
The big AI news in the past few days is the leak of the EU’s draft rules governing AI. Politico covered the leaked draft, and here’s a good thread on it:

I’ll give you my takeaway from this document up-front: These rules amount to a decision by the EU to just not have an AI startup ecosystem and to let a handful of BigCos dominate all AI in the region.
Seriously, you’d have to be crazy to do an AI-based startup in the EU once these rules go into effect. Large firms that have the legal resources to navigate these rules (and game them) will be fine, but smaller startups would be walking into a death trap.
In respect of the fact that it strongly favors large, established companies over a startup ecosystem, these rules are very much like GDPR.
As for my other thoughts on the rules, as I read through them I noticed a few main issues that kept coming up.
First, key definitions are vague. As was pointed out in the thread above, concepts like “high-risk AI” or even just “AI” itself are vague and possibly over-broad in the document.
Another example is “fairness,” which comes up in the doc a bit. People are currently fighting about basic notions of what “fairness” looks like, and the document seems to be trying to avoid taking a clear stand on that. This is consistent with the AI ethics literature I’m reading, which typically mentions competing fairness definitions and then refrains from picking a side.
Even where a definition of a key concept is attempted, as with the below list of “harms” from “high-risk AI systems,” the specifics are often pretty broad:
Items (b) and (d) above strike me as exceptionally vague. Or, maybe it would be better to say that I’m sure there are many things that are currently legal in the EU that would run afoul of these two. But perhaps there’s a way that, say, credit scores and standardized testing do not run afoul of (d) in the way some machine learning application would, and I’m just not seeing it.
The other big problem with this document is that philosophical problems are mistaken for technical problems. Check out this section:
Users should be able to understand and control how the AI system outputs are produced. High-risk AI systems should thus be accompanied by relevant documentation and instructions of use and include concise, clear and, to the extent possible, non-technical information. This information should specify, in particular, the identity and contact details of the provider of the AI system, the capabilities and limitations of the AI system, its general logic and underlying assumptions, mitigating or precautionary measures, which shall be taken by users, and the expected lifetime of the AI system and any necessary maintenance and care measures.
A lot of the above is exactly like asking for a magic algorithm that can tell “fake news” from “real news.” When you say you want users to understand how and why the AI gets from certain inputs to certain outputs, you’re asking for a level of transparency that we just don’t have and probably won’t get.
Even worse, as models get larger and more powerful, explainability gets further away from us.
As for what we should do in the US, in a previous post, I suggested the SEC as a possible model for regulating AI. But reading through this mess of rules, it occurs to me that maybe we should just not ever have a centralized regulatory apparatus for AI and machine learning.
In place of an attempt to “regulate AI,” what if we just let each area of our society — healthcare, the judicial system, the financial system, etc. — regulate the uses of AI within its own purview?
For instance, we don’t have a centralized “database regulation” regime, but in healthcare we have HIPAA and in other areas we have certain privacy regulations that touch on databases. I suspect this may be the correct model for AI/ML.
TSMC and a potential Chinese invasion of Taiwan
By way of follow-up to my previous post on the semiconductor market dynamics surrounding a potential Chinese invasion of Taiwan, there have been even more developments on this front. Here’s supply chain expert @man_integrated with a solid summary of the recent tit-for-tat:

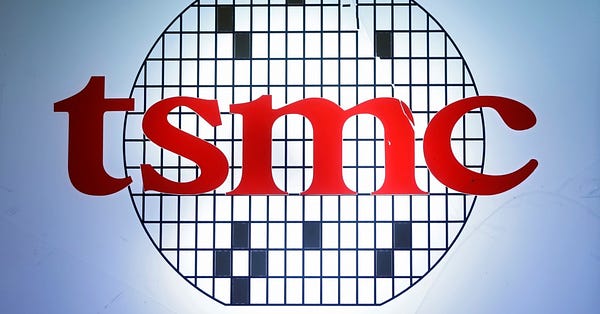
In further cutting off PRC from TSMC’s fabs, I fear we are actually lowering the bar for invasion. My logic is this:
My previous post argued that an invasion of Taiwan would mean the end of TSMC, and the end of TSMC would be worse for China than for everyone else. So China losing access to TSMC would be a reason not to invade.
But if China loses access to TSMC anyway, then the threat of that loss of access stops being a barrier.
Given how screwed China’s semiconductor ambitions would be if they invade and TSMC ceases to exist, it seems to me to be better for Taiwan and everyone else if things go in the opposite direction from where we’re driving them, i.e. if China becomes more dependent on TSMC, not less.
Maybe I have this all wrong, though, and am missing some wrinkle.
Global chip shortage has similarities to firearm & ammo shortages
Speaking of TSMC and sanctions, it’s one of a group of chipmakers whose execs have recently warned that the current chip shortage will likely stretch out into 2023.
This shortage has its roots in pandemic-related supply chain disruptions and natural disasters, but it shares one driver that I’ve also seen in my reporting on politically driven gun and ammo panic buying. Here’s the relevant part of the WaPo coverage:
One problem complicating supply at the moment: Manufacturers are placing chip orders with multiple factories because they aren’t sure which orders will come through, said Willy Shih, a Harvard Business School professor who specializes in technology and manufacturing.
“Imagine you’re an automaker and you want more of a chip and you are being quoted a lead time of a year. How many are you going to order? Are you going to order from multiple sources? You bet [you are],” Shih said.
The chaotic ordering is making it harder for chipmakers to understand where they need to allocate supply to meet real, short-term needs, he said.
Huawei actually blames the entire chip shortage on this type of hoarding and panic buying, and suggests that the US sanctions mentioned above are at fault for it:
“Because of the U.S. sanctions against Huawei, we have seen panic stockpiling among global companies, especially the Chinese ones. In the past, companies were barely stockpiling, but now they are building up three or six months’ worth of inventory ... and that has disrupted the whole system,” Rotating Chairman Eric Xu said at the company’s 18th Huawei Analyst Summit.
Interestingly enough, all of these dynamics were at work in the AR-15 market during the post-Sandy Hook buying panic. I reported on this from SHOT Show for WIRED, where gun buyers were buying up everything in sight because they were worried about losing access (due to a potential gun ban), and the gun makers I interviewed were complaining about the problem of phantom order flow as in the WaPo quote above.
Phantom order flow works like this: Would-be gun buyers would put in orders for the same gun with multiple makers, intending to take delivery of only the first rifle and then cancel the others. So there was an apparent demand surge that was many times larger than the actual demand surge. This made forecasting impossible since the makers had no idea how much of their order backlog was real vs. how much would evaporate as the panic eased.
One of the results of this activity was a set of overly pessimistic predictions about when orders would be filled. I heard from some makers that they were two or three years behind on orders, but a year out from that prediction it turned out they were back to normal. Let’s hope we’re similarly surprised to the upside on semiconductor demand.
The chip shortage may influence ML design
I think it’s possible that the GPU supply crunch may force a lot of innovation in the area of optimizing ML algorithms for different types of architectures, and for using less resources in general.
There’s a new paper out on an acceleration technique for deep learning aimed at making it more feasible to move computation back onto the CPU.
This technique has some limitations as far as model architecture, and the CPU still doesn’t beat the GPU, but this is a step in the right direction. We’ll probably see more of these kinds of optimization efforts as the chip shortage drags on.
Twitter launches Responsible Machine Learning initiative
Twitter’s cropping algorithm is one of the stock examples of “racist AI” in the literature, up there with the Google Photos gorilla scandal. In that context, it’s not a surprise the image cropping algo features prominently in their announcement of their new, company-wide initiative:


As I pointed out on Twitter, embedded in this announcement is a social justice paradox that they are just not going to be able to solve:
The problem they face is highlighted by a user in a tweet responding to the announcement:

As I’ve mentioned a few times in this newsletter, the AI ethics crowd is constantly protesting that it’s inherently problematic to ever use ML to infer gender, sexual orientation, race, emotional states, or any other characteristic that falls under the increasingly broad umbrella of “self-identification.”
Hence the paradox: You can’t improve what you can’t measure, so if you’re not allowed to measure race or gender distributions in a dataset, then how can you improve it with quotas and targets?
There is no fix for this because self-ID doesn’t scale. You can’t make equity-oriented decisions on a dataset of hundreds of millions of users without automated inference of protected characteristics unless you can somehow get all of those users to divulge those characteristics to you. But then that conflicts with privacy desiderata. There’s no engineering fix for this that makes all sides happy.
You also can’t target ads at what you can’t measure. None of these companies are going to just refuse to do this kind of inference— they’ll just do it secretly and use it for ad targeting, and people will complain about it and they’ll deny it’s happening. Capitalism will win this fight, so to get their way they’ll have to go after capitalism… which is a thing that’s very much happening: