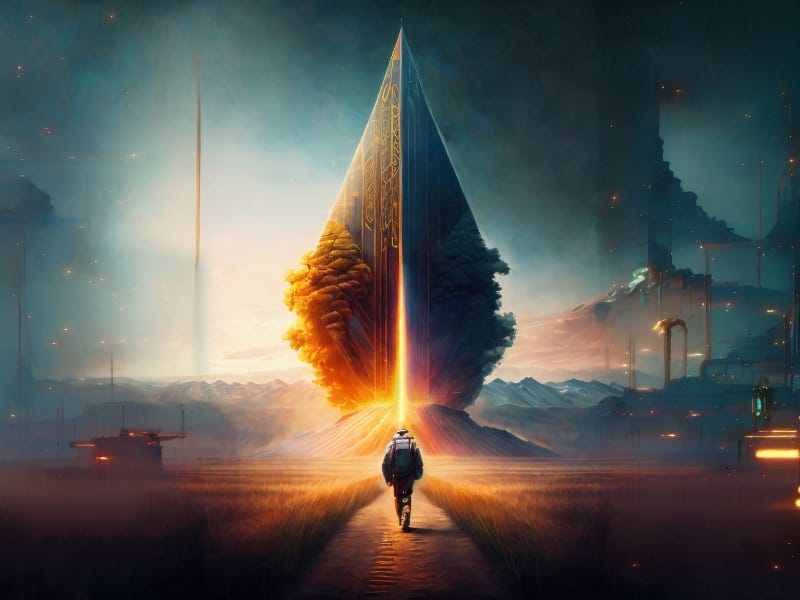
I’ve been doing some writing on AI topics around the web, and I want to make sure those of you in my newsletter audience are able to follow any work I do on other platforms. So periodically I’ll send out one of these “Elsewhere” posts to point you to the other posts.
⚡️First up is an in-depth piece I did on machine learning and the future of programming: The Fourth Age Of Programming. This is a large post with diagrams and such, similar to my Stable Diffusion posts in terms of quality and detail. So if this is the kind of content you signed up to this newsletter for, then you’ll want to check it out.
I’ve pasted in the opener below, but you’ll need to hit the Replit blog for the full post:
The technological singularity—or simply the singularity—is a hypothetical future point in time at which technological growth becomes uncontrollable and irreversible, resulting in unforeseeable changes to human civilization. According to the most popular version of the singularity hypothesis, I.J. Good's intelligence explosion model, an upgradable intelligent agent will eventually enter a "runaway reaction" of self-improvement cycles, each new and more intelligent generation appearing more and more rapidly, causing an "explosion" in intelligence and resulting in a powerful superintelligence that qualitatively far surpasses all human intelligence. — Wikipedia
In normieland, where I still spend plenty of time both online and IRL, software’s newfound ability to write, draw, and speak like we humans is often taken as evidence that the machines are about to remake our entire society (again) and totally change the nature and value of labor (again). I don’t think the normies are wrong about this, but as flashy as Stable Diffusion and ChatGPT are, old heads know that the Robot Apocalypse has exactly one and only one horseman: computer programs that can write computer programs.
Artificially intelligent machines can’t ascend to godhood via the long-prophesied runaway spiral of continuous self-improvement until we invent a machine learning model that can code a better version of itself. We’re not there yet, and it’s not clear how far away such a development is, but as of the mid-2021 release of OpenAI’s Codex model, we’re a lot closer than we were just a few years ago.
Given that self-improving computer programs would likely be the most important human invention since writing, there is no other part of the AI content generation revolution that’s worthy of more study and careful scrutiny than generative models that output code.
And of all the new ML-powered programming offerings in this growing ecosystem, Replit has the tools I’m watching most closely. No other programming platform is in a position to train models on a dataset that includes the following from legions of programmers and millions of projects:
Real-time keystroke and clickstream data
Detailed execution and performance data
Character-level file changes
If you were going to design a platform for the express purpose of teaching machines to code, it would probably be a cloud-hosted IDE plus execution environment that looks a lot like Replit. So while most of what you’ll read in this article and followups is applicable to all AI code generation tools more generally, I’ll be focusing on Replit’s toolset because right now it’s the richest and most advanced, and has the most potential for advancing the state-of-the-art.
[Read the rest of this article at Replit…]
👉 The other piece I recently did on AI was a lot shorter — a quick piece about the possible relationship between Stable Diffusion and revenge porn. Here’s a link to the Twitter thread where I promoted it: