Computer vision, AI in war, and the definition of "bad"
A roundup of work, mostly bad, but with bright spots
I’m trying a new thing with this post where I try to work my way through my links backlog in Notion, and write commentary on the more interesting ones. This helps me stay on top of where AI is headed, and I hope it helps you, as well.
I should also note that a lot of the work I'm reviewing in this post is just plain bad — poorly written, sloppily argued, full of holes, or just journalism or commentary passing as “scholarship.” I think it’s important to call out bad work, despite the fact that you’ll be slandered for it as some flavor of “alt-right” when you do. Because if you don’t call out bad work then it will just take over your discipline.
New computer vision paper shows field is headed in the wrong direction
The computer vision community is evolving, and not everyone is happy about it because not all of the changes are good. Computer vision is an example of an area of tech that has thus far avoided the worst of the culture wars that have riven other disciplines, but if a new paper is any indication, it is sliding into the same politics-riddled territory as the rest of the world.
Recently, a pair of researchers undertook a formal investigation of how the field’s members feel about the recent changes. They asked 56 students, professors, and industry researchers to submit a piece of prose and fill out a survey about their subjective experience of the field of computer vision, and then they summarized all the responses a paper.

If this doesn’t quite sound like hard-hitting science, that’s because it isn’t. And the authors kind of admit it in the paper:
We are well aware taht this is not a typical CVPR paper: we beat no benchmarks, we introduce no datasets, we present no novel loss functions. But we argue that this type of work is nevertheless of central importance to CVPR, not to be sidelined off its main track. We identify how the growth of computer visioned has affected a diverse range of individuals: these affects cannot be measured in terms of aggregate quantitative metrics. This paper seeks to amplify these emotions...
Yeah, you read that right: the goal is the “amplify emotions.” The authors’ social media feeds are filled with the standard identity politics causes, and it seems pretty clear they want to drag their field in this trendy direction.
One UCLA professor called it, “The first humanities-oriented CVPR paper I have ever seen.” Guy, speaking as a former humanities person, that is not a compliment.
PSA for computer vision experts: If you don’t want this kind of feelings-oriented work to take over your discipline, push back on this paper — question its value. Because this is the camel’s nose under the tent. If this is allowed to stand, more will come.
Having said that, I do think there’s a place for this kind of feelings-focused investigation, and that place is the popular tech press. If a reporter had done the kind of data collection and write-ups that went into this paper, that would be a worthwhile article. As an editor, I’d totally assign that.
What bothers me is that this is being presented as serious academic work, and not as the popular science journalism it is.
This business of trafficking in feelings and stereotypes is part of a growing trend I’ve been monitoring in AI/ML circles. A recent paper by the Mozilla Foundation, for instance, contained a whole section that was nothing but totally unsourced, unsubstantiated “techbro” stereotypes. In fact, the main stereotype referenced in their title appears in the paper without a single citation or example — it’s just dropped into the paper without a shred of evidence. Remarkable.

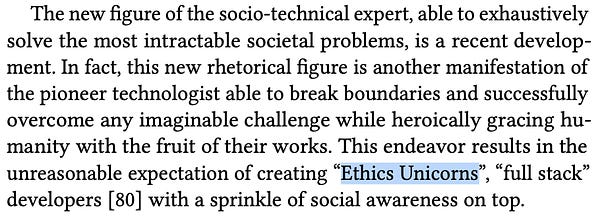

Moving on to the actual contents (vs. the optics) of this latest paper on computer vision, it’s an interesting document. Some of the findings square with my own reporting in some ways. Here’s a summary:
Too many papers: The authors repeat a complaint I’ve heard a number of times from people in ML, which is that there are now just too many papers for anyone to stay on top of. This dynamic is behind a lot of the other complaints in the paper.
Ethics concerns: A lot of the computer vision ML work is around drawing inferences from the way people look or act — a big no-no right now. Many of the FATML folks argue that tasks that attempt to infer interior states like race, gender, or sexual orientation from images are inherently wrong and shouldn’t be done.
The deep learning (DL) revolution: The other main factor behind many of the changes participants identified, is the past few years’ revolution in computer vision brought about by the rise of deep learning in the past decade. The whole field has been taken over by deep learning, and it’s exciting in some ways (magical results) and boring in others (DL is all anyone wants to do).
Less theory, more application: This one is really fascinating to me because it’s about how some of the researchers got into the field in order to learn something fundamental about the machinery of human perception, but they find they’re now toiling away at specialized applications that solve very niche problems with little hope of generalizing the results to shed light on larger questions.
One question I’d ask these folks: what if the human visual system is a giant collection of such specialized, task-specific systems that operate at various levels of abstraction? What if there’s, say, one quasi-general system for detecting bilateral symmetry, and another slightly less general one for detecting threat displays, and yet another very niche one that specializes solely in detecting snakes? What if very little of our visual system is generalizable?
I do think one of the respondent’s warnings about how the “magic” of DL may be warping the field in various ways — all the applications are increasingly commercial, and the students are more interested in throwing DL at a problem and moving on — is something to keep an eye on. This does happen — when a way of working in a field is both socially rewarded and pretty easy to do without really thinking critically, then that kind of work tends to proliferate at the expense of everything else.
Indeed, I’d turn that warning back on the paper’s authors themselves as follows: when you come across a framework for doing “scholarship” that’s socially rewarded, trendy, and a lot easier to do than... how did you put it? — “beat[ing] benchmarks... introduc[ing] datasets... present[ing] novel loss functions” — see that you don’t end up just doing a bunch of that type of “scholarship” instead of the hard work of advancing the field.
People who get clout from being tired online are tired in surveys: Given that they reached out to various activist subcommunities whose members spend a lot of time doing “I’m so exhausted” on Twitter, it’s no shocker to me that some respondents reported being “tired” because of all the -isms in the field. When I saw the methodology section earlier in the paper, I definitely put “I’m so tired,” “gaslighting,” and “most ML tasks are problematic and should not exist” on my bingo card. I was not disappointed.
Kids these days: This cracked me up: “P19 tells of feeling helpless when students are unable to comprehend a classic paper ‘on spatio-temporal interest points... the student spent more than a week and returned completely puzzled by the paper written in the dinosaurs era’ before deep learning.” To the paper’s authors: if you want this problem to get even worse, keep publishing popular journalism pieces dressed up as science!
AI/ML links I’m reading: AI in war, defining “bad,” diversity
Pervasive Label Errors in Test Sets Destabilize Machine Learning Benchmarks, April 2021: Much has been made of the “problematic” mislabelings in popular image datasets and the ethical harms that arise from such. But this is the first paper I’m aware of that seeks to quantify the actual performance impact of mislabelings.
In the abstract, they write: “On ImageNet with corrected labels: ResNet-18 outperforms ResNet-50 if the prevalence of originally mislabeled test examples increases by just 6%. On CIFAR-10 with corrected labels: VGG-11 outperforms VGG-19 if the prevalence of originally mislabeled test examples increases by just 5%.”
What this basically means is that smaller, less-resource-intensive models will outperform larger, costlier ones if their data is of higher quality. The mislabelings are costing everyone money.
Introduction to ethics in the use of AI in war, February 2020: I really don’t know why this Abhishek Gupta wrote such a long piece on this topic when his take is essentially that war is bad and AI researchers shouldn’t be enabling it.
He writes: “Perhaps, a clarion call from researchers and practitioners in refusing to work on applications that have a strong likelihood to be reused in warfare can give us more time while we figure out both the governance and technical measures that can allow for safe use.”
I have questions for Gupta and everyone else who would demand that AI researchers refuse to work on so-called “dual-use” technologies:
Given that there’s another AI superpower in the world that is aggressively developing military applications for ML, aren’t you essentially calling for our researchers to unilaterally disarm the West?
Who gave you the right to call for the West’s unilateral disarmament? I know I sure didn’t get a vote there.
Have you thought through what happens if western AI researchers follow your advice to stand down, and cede military AI dominance to the Chinese? Is a world where China dominates everyone else militarily fairer or more just than a world where Western nations are at parity with China?
In general, for all the sprinkling of the word “ethics” in the title and headings of this piece, the actual ethical reasoning in it is not at all clear or sophisticated.
Discriminating Systems: Gender, Race, and Power in AI, April 2019: I did a Twitter thread on this paper, and the take-home is this: when it comes to “diversity” in AI and STEM in general, it seems that Asians don’t count towards any type of diversity score.
This paper is a really egregious example of the standard Asian erasure in action, where “diversity” is narrowly defined as black and latino representation. Indians, Chinese, Filipinos, and all other non-white groups that don’t fall into the “black” and “latino” categories are just erased in this paper.
In addition to the Asian erasure, it’s just shoddy work. This report claims to be the result of a year-long study on “diversity in AI,” yet they have zero actual data on the racial or gender makeup of private-sector AI research teams. None. They try to back into this data from company-wide diversity statements and tech press analyses of conference paper submissions, but there is no real survey or study data that bear directly on the report’s core “diversity in AI” interest.
This is not quality, careful scholarship, and if you’re in a field where people are producing work like this then you should push back on it. Otherwise, you’ll see more like it.
In AI ethics, “bad” isn’t good enough, December 14, 2020: This is a great blog post, and it gets at something I complain about all the time: the handwaving and general imprecision around definitions of “harm” in discussions of AI ethics.
This is just really excellent:
It would be a mistake to read an article about painful stitches and to conclude that we should no longer carry out surgeries. And it would be a mistake to read an article about a harm caused by an AI system and conclude that we shouldn’t be using that AI system. Similarly, it would be a mistake to read an article about a benefit caused by an AI system and conclude that it’s fine for us to use that system.
Drawing conclusions about what we have all things considered reasons to do from pro tanto arguments discourages us from carrying out work that is essential to AI ethics. It discourages us from exploring alternative ways of deploying systems, evaluating the benefits of those systems, or assessing the harms of the existing institutions and systems that they could replace.
This is why we have to bear in mind that in AI ethics, “bad” often isn’t good enough.
Read the whole thing.